Traceable machine vision systems for digital industrial applications
23IND08 DI-Vision
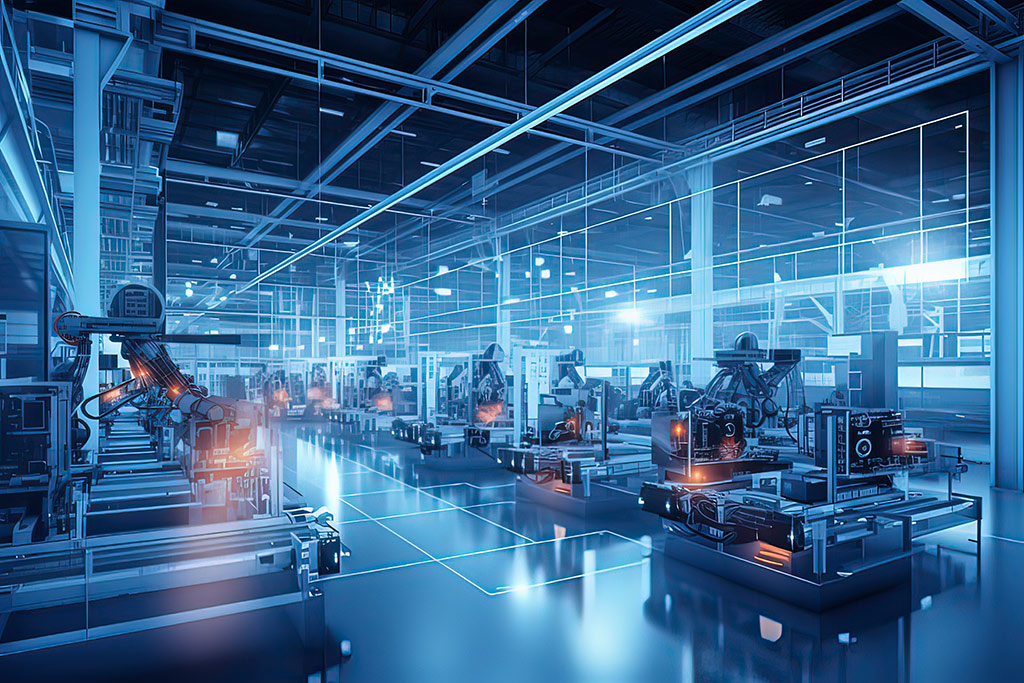
Industry 4.0 aims to transform industrial processes through the integration of ‘smart’ technologies such as AI, automation, and robotics, into manufacturing processes. Integral to many high-value industries are machine vision systems (MVSs) where cameras and sensors are used to monitor production. These systems enable correct part placement during assembly, coordinate robots and detect defects whilst improving efficiency, increasing productivity, and reducing costs. The integration of ‘digital twins’ could further improve MVSs by providing virtual models of physical objects that use real-time data from sensors to simulate MVSs behaviour and monitor operations. However metrological standards, calibration methods, traceability and uncertainty assessment for existing and newly developed MVSs are lacking.
Machine vision systems are crucial to many high-value industries, where Europe is globally competitive, and to the EU objectives for digital transformation and the Green Deal. However, further work is needed to develop digital twins for these systems based on data and physical driven models and the implementation of robust matching and evaluation algorithms for recorded raw data. This will enable the accurate classification of defects or the extraction of geometrical parameters from the surface. In addition, traceability for these systems is also required. The tools developed in this project, combined with uncertainty evaluation methods, will be applied throughout industrial applications on macro‑ and microscale specimens in order to reach better accuracy, productivity and flexibility as well as improved safety and cost efficiency.
This project will generate traceability for the Machine Vision Systems (MVSs) that are deployed in industrial applications including macro- and microscale products based on the development of traceable material standards, V-DTs (virtual digital twins), uncertainty evaluation methods, robust and reliable dense-image matching algorithms, as well as robust classifiers and analysing algorithms.
The project (23IND08 DI-Vision) has received funding from the European Partnership on Metrology, co-financed from the European Union’s Horizon Europe Research and Innovation Programme and by the Participating States.
Funded by the European Union. Views and opinions expressed are however those of the author(s) only and do not necessarily reflect those of the European Union or EURAMET. Neither the European Union nor the granting authority can be held responsible for them.